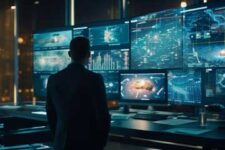
Beyond the Numbers: The Impactful Role of Data Science in Business
Data science has emerged as a game-changer in the dynamic terrain of modern business, where competition is strong and markets evolve at an unprecedented rate. Data science is no longer limited to research and development; it now pervades every aspect of corporate operations, from marketing and sales to finance and strategic planning. This investigation delves into the influential role of data science in influencing the business landscape, going beyond the mere interpretation of numbers to drive innovation, efficiency, and strategic decision-making.
Business Analytics’ Evolution
Before delving into the present status of data science in business, it’s critical to understand the history of business analytics. Historically, business decisions were frequently influenced by intuition, experience, and historical tendencies. However, as the volume of data provided by organizations increased, it became clear that more complex analytical tools were required.
The introduction of business intelligence technologies signaled a fundamental shift. These tools enabled firms to collect and evaluate historical data, providing insights into previous performance. While important, these insights were frequently retrospective, providing a glance back rather than a look forward.
Here comes data science. Data science, as opposed to traditional business analytics, uses advanced statistical approaches, machine learning algorithms, and predictive modeling to identify patterns, make forecasts, and extract actionable insights. This paradigm shift has not only improved decision-making accuracy, but has also enabled firms to foresee future trends and adapt proactively to shifting market dynamics.
Using Predictive Analytics to Drive Innovation
The potential to drive innovation through predictive analytics is one of the most important contributions of data science to business. Businesses can estimate future trends and consumer behavior by examining historical data and recognizing patterns. This foresight enables businesses to reinvent products, services, and strategies in response to market demands.
Take the retail industry, for example. Predictive analytics is used by e-commerce platforms to assess client browsing and purchasing behaviors. These technologies can personalize recommendations, optimize inventory management, and improve the overall shopping experience by understanding what customers are likely to buy in the future.
Data science is useful in guiding innovation in the field of product development. Businesses can discover areas for development, prioritize features, and modify product offers to fit changing consumer preferences by using sentiment analysis of client feedback. This continual process of innovation, driven by data-based insights, has become a hallmark of digital-age success.
Data-Driven Efficiency for Operational Optimization
The lifeblood of any successful firm is efficiency, and data science serves as a catalyst for refining operational procedures. Data-driven insights enable firms to streamline operations, decrease costs, and improve overall efficiency across the supply chain, production, and logistics.
Data science, for example, is critical in demand forecasting in supply chain management. Businesses can manage inventory levels, minimize stockouts, and reduce excess inventory carrying costs by assessing historical sales data, market patterns, and external factors such as weather and economic indicators. This not only increases profits but also assures that products are available when and where customers need them.
Similarly, predictive maintenance fueled by data science has become a game changer in manufacturing. Businesses can predict when machinery is likely to break and arrange maintenance in advance by monitoring equipment performance and analyzing data from sensors. This not only reduces downtime but also increases the lifespan of important assets, resulting in significant cost savings.
Customizing Customer Experiences
Customers have grown to expect individualized experiences in an age of information overload. Data science is critical to satisfying this expectation since it enables firms to understand their clients on an individual level and adjust interactions accordingly.
Personalization is visible in a variety of business sectors. Machine learning algorithms are used in e-commerce to fuel recommendation engines, which assess a user’s prior behavior, preferences, and purchase history to propose things they are likely to be interested in. This not only improves the shopping experience for customers, but it also promotes sales through targeted marketing.
Data science is fueling the era of hyper-targeted advertising in the world of digital marketing. Businesses may design highly tailored marketing campaigns that resonate with certain audience segments by evaluating demographic data, internet behavior, and social media participation. This tailored strategy not only improves marketing efficacy, but also increases customer engagement and brand loyalty.
Predictive Modeling for Risk Mitigation
Businesses work in an uncertain and dangerous climate. Data science provides a broad toolkit for risk mitigation through predictive modeling and analysis, whether it’s financial markets, supply chain disruptions, or cybersecurity risks.
Predictive modeling, for example, is useful in credit rating in finance. Predictive models assess the chance of default by examining an individual’s credit history, financial behavior, and other pertinent indicators, allowing lenders to make informed judgments about issuing loans. This not only protects lending institutions’ financial soundness, but also assures fair and data-driven lending practices.
Natural disasters, geopolitical upheavals, and economic downturns can all have a significant impact on supply chains. Organizations can use data science to identify and quantify these risks, enabling proactive risk management techniques. Businesses may construct contingency plans, diversify suppliers, and build resilience against unforeseen interruptions by modeling alternative scenarios and their possible impact on the supply chain.
Boardroom Strategic Decision-Making
One of the most transformational aspects of data science in business is its role in shaping high-level strategic decision-making. Boards and executive teams today rely on data-driven insights to develop goals, allocate resources, and lead the organization’s general direction.
Strategic planning, which was originally relied solely on intuition and industry knowledge, is now aided by a wealth of data. Data science enables firms to undertake market analysis, examine competition landscapes, and find growth possibilities with previously impossible precision.
Furthermore, data-driven decision-making extends beyond strategic planning to encompass all aspects of corporate governance. Businesses use data science to monitor key performance indicators (KPIs), track progress, and course-correct in real-time, from setting performance targets to evaluating the efficacy of marketing efforts.
Using Data Science to Navigate Regulatory Compliance
Businesses face an expanding number of compliance responsibilities in an era of increased regulatory scrutiny. Data science emerges as a critical ally in navigating this complicated terrain, assisting firms in complying with rules, managing risks, and upholding ethical norms.
Data science is critical in guaranteeing compliance in industries such as healthcare and finance, where rules are particularly severe. Machine learning models can examine massive information to detect anomalies or potential regulatory violations. This proactive strategy not only protects against legal ramifications, but also fosters trust among stakeholders and customers.
Data science also contributes to ethical considerations in company operations. It allows companies to monitor and enforce fair practices, ensuring that algorithms and models do not unintentionally perpetuate biases or discriminate against specific populations. This dedication to ethical data practices not only complies with regulatory standards, but also builds a reputation for integrity and accountability.
Data Democratization: Empowering Every Department
As the effect of data science on business grows, there is an increasing tendency towards data democratization. Data-driven insights are no longer the sole realm of data scientists and analysts; they are becoming more available to personnel across all departments within a business.
Self-service analytics tools enable non-technical people to freely explore and evaluate data. Marketing teams can examine campaign performance, HR departments can track employee engagement, and sales teams can estimate revenue—all without specific data science knowledge.
This data democratization not only improves operational efficiency, but it also develops a data-driven culture within enterprises. Decision-making becomes more flexible, informed, and sensitive to the dynamic demands of the business environment when workers at all levels have access to data and can extract insights.
Future Challenges: Ethical Considerations and Bias Mitigation
While data science’s impact on business is clearly favorable, it is not without obstacles. Ethical concerns, particularly those related to privacy and bias, have risen to the fore in conversations about data-driven decision-making.
The collecting and use of enormous volumes of personal data raises privacy concerns. Businesses must strike a fine balance between using data to get insights and protecting individuals’ privacy rights. GDPR (General Data Protection Regulation) regulatory frameworks in Europe emphasize the significance of ethical data practices and honest communication with consumers about the use of their data.
Another major issue is algorithm bias. Machine learning algorithms trained on historical data may perpetuate existing biases and produce discriminating results. Identifying and mitigating biases in algorithms requires a dedicated effort at every stage of the data science lifecycle, from data collection to model training and deployment.
AI Integration and Continuous Innovation on the Horizon
With the integration of artificial intelligence (AI) and the constant pursuit of continuous innovation, the importance of data science in business is poised to expand even more in the future. AI, powered by machine learning and deep learning algorithms, has the potential to push automation, personalization, and efficiency to new heights.
Chatbots powered by AI are becoming increasingly smart in customer service, giving quick solutions to customer queries and even predicting their needs. Natural language processing and machine learning are used by virtual assistants to interpret user requests, learn from interactions, and improve the quality of help over time.
Another sector where data science will play a critical role is the Internet of Things (IoT). The volume of data created by IoT devices will soar as they become more networked. Data science will be critical in gaining useful insights from this flood of data, allowing businesses to streamline operations, improve user experiences, and drive innovation.
Furthermore, the focus on continual innovation in data science approaches and technologies will continue to be a driving force. The future promises a tapestry of possibilities that will transform how businesses leverage the power of data, from advances in explainable AI to the research of quantum computing for data analysis.
Sustainability and Social Impact: A New Data Science Frontier
The convergence of data science, sustainability, and social impact is becoming more obvious as corporations embrace their responsibility as global citizens. Data-driven approaches are being used to address some of the world’s most important issues, including climate change and environmental protection, as well as social justice and healthcare access.
Data science is used in supply chain management to track the environmental impact of products, find sustainable sourcing strategies, and lower the carbon footprint of activities. Businesses are increasingly adding sustainability measures into their decision-making processes in order to reconcile economic goals with environmental and social responsibilities.
Data-driven insights can also help social impact efforts. Data science may help guide policies that improve access to education, healthcare, and economic opportunity. Data science is a strong tool for creating positive change, whether it’s optimizing resource allocation in underprivileged communities or anticipating disease outbreaks for preventive intervention.
To summarize
As we go across the revolutionary environment of data science in business, it becomes clear that its impact goes far beyond numbers. Data science, beyond algorithms and analytics, is a driver of innovation, efficiency, and strategic evolution. It is a compass that guides businesses through the intricacies of the digital world, allowing them to derive actionable insights that create the future rather than just read numbers.
The path of data science in business is a never-ending story distinguished by constant innovation, ethical considerations, and a commitment to using data to make a beneficial impact. As we stand at the crossroads of technology and human inventiveness, the role of data science in business continues to evolve, guiding enterprises toward a future where data-driven decisions are synonymous with long-term success.